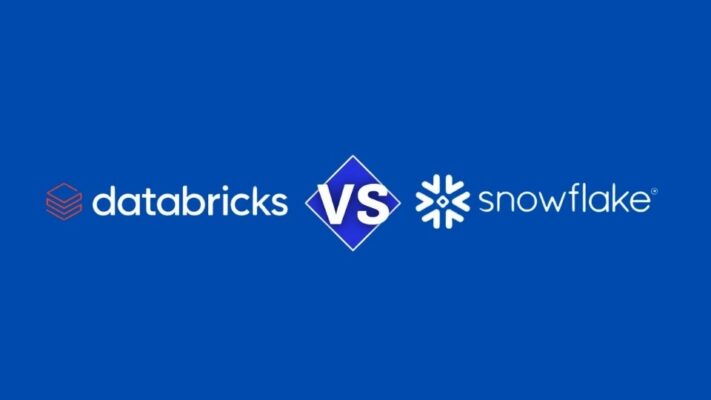
In the ever-evolving landscape of big data and cloud computing, two platforms have emerged as frontrunners: Databricks and Snowflake. The “Databricks vs Snowflake” debate has become increasingly relevant for businesses seeking to optimize their data infrastructure and analytics capabilities. This comprehensive guide will delve into the intricacies of Databricks vs Snowflake, exploring their features, strengths, weaknesses, and use cases to help you make an informed decision for your organization.
Introduction to Databricks vs Snowflake
The Databricks vs Snowflake comparison has become a hot topic in the world of big data and cloud analytics. Both platforms offer powerful solutions for data warehousing, analytics, and machine learning, but they approach these challenges in different ways. Understanding the nuances of Databricks or Snowflake is crucial for organizations looking to leverage their data assets effectively.
Overview of Databricks
Databricks, founded by the creators of Apache Spark, is a unified analytics platform that combines the power of data engineering, data science, and business analytics. It provides a collaborative environment for data teams to work on large-scale data processing and machine learning projects.
Overview of Snowflake
Snowflake is a cloud-native data warehouse platform that offers a unique architecture separating compute and storage. It’s designed to handle diverse data workloads, from data warehousing to data lakes and data applications.
Databricks vs Snowflake: Architecture Comparison
When comparing Databricks vs Snowflake, one of the key differences lies in their architectural approaches:
– Databricks: Built on Apache Spark, Databricks uses a distributed computing model with a unified data analytics platform.
– Snowflake: Employs a unique multi-cluster, shared data architecture that separates compute and storage.
The Databricks and Snowflake architectural differences impact how each platform handles data processing, scalability, and performance.
Databricks vs Snowflake: Performance Analysis
In the Databricks vs Snowflake performance showdown:
– Databricks excels in processing large-scale, complex data workloads, particularly for data science and machine learning tasks.
– Snowflake shines in traditional data warehousing scenarios, offering impressive query performance for structured data.
The Databricks or Snowflake performance comparison often depends on the specific use case and data types involved.
Databricks vs Snowflake: Scalability and Elasticity
Both platforms offer robust scalability, but their approaches differ:
– Databricks leverages Spark’s distributed computing model for horizontal scalability.
– Snowflake’s multi-cluster architecture allows for independent scaling of compute and storage.
In the Databricks vs Snowflake scalability contest, both platforms demonstrate strong capabilities, with Snowflake potentially having an edge in rapid elasticity for varying workloads.
Databricks vs Snowflake: Data Processing Capabilities
The Databricks vs Snowflake comparison in data processing reveals:
– Databricks offers superior capabilities for complex data transformations and ETL processes, thanks to its Spark foundation.
– Snowflake provides excellent support for SQL-based data transformations and analytics on structured and semi-structured data.
The choice between Databricks and Snowflake for data processing often depends on the complexity of your data pipelines and the skills of your team.
Databricks vs Snowflake: Machine Learning and AI Integration
In the realm of machine learning and AI:
– Databricks has a clear advantage with its integrated MLflow for managing the machine learning lifecycle and support for various ML frameworks.
– Snowflake has been expanding its ML capabilities but generally relies more on integrations with external ML tools.
The Databricks vs Snowflake comparison in ML and AI typically favors Databricks for organizations heavily focused on advanced analytics and data science.
Databricks vs Snowflake: Pricing Models
The Databricks vs Snowflake pricing comparison is complex due to their different models:
– Databricks uses a consumption-based model with separate charges for compute and Databricks Units (DBUs).
– Snowflake employs a credit-based system where customers purchase credits that are consumed based on usage.
When evaluating Databricks and Snowflake costs, organizations need to consider their specific usage patterns and requirements.
Databricks vs Snowflake: Security and Compliance
Both platforms offer robust security features:
– Databricks provides end-to-end encryption, access controls, and compliance with various industry standards.
– Snowflake offers similar security capabilities, including encryption at rest and in transit, role-based access control, and compliance certifications.
In the Databricks vs Snowflake security comparison, both platforms are strong contenders, with specific features that may appeal to different organizational needs.
Ease of Use and User Interface
The user experience is an important factor in the Databricks or Snowflake decision:
– Databricks offers a collaborative notebook interface that’s popular among data scientists and analysts.
– Snowflake provides a more traditional SQL-based interface that’s familiar to database administrators and analysts.
The Databricks and Snowflake usability comparison often comes down to the preferences and skills of your team.
Databricks vs Snowflake: Ecosystem and Integrations
Both platforms offer extensive ecosystems:
– Databricks integrates well with the broader Apache Spark ecosystem and various data science tools.
– Snowflake has a wide range of partner integrations, particularly with BI and ETL tools.
The Databricks vs Snowflake ecosystem comparison may favor one platform depending on your existing technology stack and integration requirements.
Use Cases and Industry Applications
The Databricks vs Snowflake comparison varies across different use cases:
– Databricks is often preferred for advanced analytics, real-time processing, and machine learning workflows.
– Snowflake excels in traditional data warehousing, business intelligence, and data sharing scenarios.
When considering Databricks or Snowflake for your industry, consider the specific data challenges and analytics requirements you face.
Pros and Cons of Databricks
Pros:
– Powerful data processing and analytics capabilities
– Excellent support for machine learning and AI workloads
– Strong collaboration features for data teams
Cons:
– Can be complex to set up and manage
– Potentially higher costs for large-scale deployments
– Steeper learning curve for non-technical users
Pros and Cons of Snowflake
Pros:
– Easy to use and manage
– Excellent performance for data warehousing workloads
– Flexible pricing model
Cons:
– Limited support for advanced analytics and machine learning
– Can become costly for high-volume data processing
– Less suitable for real-time data processing
Expert Recommendations
When deciding between Databricks or Snowflake, consider the following factors:
1. Data workload types: Choose Databricks for complex, varied data processing and ML workloads; choose Snowflake for traditional data warehousing and BI.
2. Team skills: Databricks may be preferable for teams with strong programming skills, while Snowflake might be better for SQL-focused teams.
3. Scalability needs: Both offer excellent scalability, but Snowflake may have an edge for rapidly changing workloads.
4. Budget considerations: Carefully analyze your usage patterns to determine which platform offers the most cost-effective solution for your needs.
5. Integration requirements: Consider which platform aligns better with your existing technology stack and future plans.
Future Outlook
As the Databricks vs Snowflake competition continues, both platforms are likely to evolve:
– Databricks is expected to enhance its data warehousing capabilities and ease of use.
– Snowflake is likely to expand its support for advanced analytics and machine learning.
The future of the Databricks and Snowflake landscape will likely see increased convergence in features, making the choice even more nuanced for organizations.
Conclusion
The Databricks vs Snowflake comparison reveals two powerful platforms with distinct strengths. Databricks excels in complex data processing, advanced analytics, and machine learning, making it ideal for organizations with diverse data workloads and strong data science needs. Snowflake shines in traditional data warehousing, offering ease of use and impressive performance for structured data analytics.
Ultimately, the choice between Databricks or Snowflake depends on your organization’s specific requirements, existing infrastructure, team skills, and long-term data strategy. Many organizations even find value in using both platforms for different use cases, leveraging the strengths of each.
As you navigate the Databricks or Snowflake decision, consider partnering with experts who can provide tailored advice based on your unique needs. Upcore Technologies offers comprehensive consulting services to help organizations make informed decisions about their data infrastructure and analytics platforms. With deep expertise in both Databricks and Snowflake, Upcore Technologies can guide you through the selection process, implementation, and optimization of your chosen platform to maximize the value of your data assets.
Remember, the Databricks and Snowflake debate is not about finding a one-size-fits-all solution, but about identifying the platform that best aligns with your organization’s goals and empowers you to unlock the full potential of your data.