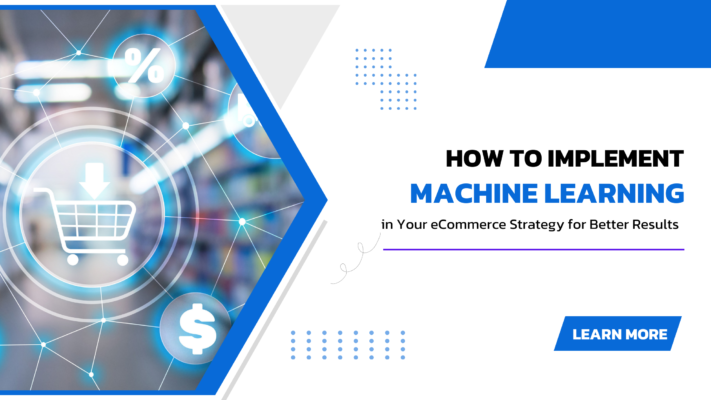
Integrating machine learning into your eCommerce strategy can significantly enhance customer engagement, optimize operations, and drive better results. This blog explores effective methods for implementing Machine Learning in Your e-commerce Strategy while offering insights and best practices to help you harness its full potential.
Understanding Machine Learning in eCommerce
Machine Learning (ML) involves using algorithms that allow systems to learn from data and improve their performance over time without being explicitly programmed. In the eCommerce sector, machine learning enables businesses to analyze vast amounts of data, identify patterns, and make informed decisions that enhance the overall shopping experience.
Key Benefits of Machine Learning in Your eCommerce Strategy
1. Personalization: Machine learning can analyze customer behavior to provide personalized product recommendations, tailored promotions, and customized content, leading to increased customer satisfaction and loyalty.
2. Enhanced Customer Insights: By analyzing customer data, machine learning tools can uncover insights into buying habits, preferences, and trends, helping businesses make data-driven decisions.
3. Inventory Management: Implementing machine learning can optimize inventory levels by predicting demand fluctuations, thereby reducing stockouts and overstock situations.
4. Fraud Detection: Machine learning algorithms can identify unusual patterns in transactions, helping to detect and prevent fraudulent activities in real-time.
5. Marketing Optimization: By analyzing campaign performance data, machine learning can assist in identifying the most effective marketing strategies, optimizing ad spend, and improving overall ROI.
Essential Components of Machine Learning in Your eCommerce Strategy
To effectively implement Machine Learning in Your eCommerce Strategy, businesses must focus on several critical components:
1. Data Collection and Management
Quality data is the foundation of any machine learning initiative. Businesses should prioritize collecting comprehensive and accurate data, including:
- Customer Demographics: Gather information such as age, gender, location, and purchase history to better understand your target audience.
- Behavioral Data: Track customer interactions on your website or app, including clicks, time spent on pages, and cart abandonment rates.
- Transaction Data: Analyze sales records, payment methods, and order details to identify purchasing trends.
Establishing a robust data management system is crucial for storing, organizing, and analyzing this data effectively.
2. Choosing the Right Machine Learning Tools and Techniques
Selecting the appropriate machine learning tools and techniques is vital for achieving desired outcomes. Here are some popular options:
- Recommendation Systems: Use collaborative filtering or content-based filtering algorithms to provide personalized product suggestions based on user behavior and preferences.
- Predictive Analytics: Employ predictive modeling techniques to forecast demand, customer behavior, and market trends. This can include regression analysis and time-series forecasting.
- Natural Language Processing (NLP): Utilize NLP techniques to analyze customer feedback, reviews, and social media interactions for sentiment analysis and trend identification.
3. Developing and Training Machine Learning Models
Building effective machine learning models requires a systematic approach:
- Define Objectives: Clearly outline the goals of your machine learning initiative, such as improving conversion rates, reducing cart abandonment, or enhancing customer retention.
- Select Features: Identify relevant features from your dataset that will contribute to the model’s predictive power.
- Split Data: Divide your dataset into training, validation, and test sets to ensure the model can generalize well to unseen data.
- Train Models: Use appropriate algorithms (e.g., decision trees, neural networks, or support vector machines) to train your models on the training dataset.
- Evaluate Performance: Assess the model’s performance using metrics such as accuracy, precision, recall, and F1-score. Adjust the model based on evaluation results to improve its effectiveness.
4. Integrating Machine Learning into Business Processes
Once the models are developed, integrating them into your existing business processes is crucial. Consider the following strategies:
- Personalization Engines: Implement recommendation engines on your website to provide personalized product suggestions in real-time based on user behavior and preferences.
- Dynamic Pricing: Use machine learning to analyze competitor pricing, demand fluctuations, and customer behavior to optimize pricing strategies and maximize revenue.
- Customer Segmentation: Leverage clustering algorithms to segment customers based on their behavior, allowing for targeted marketing campaigns and tailored experiences.
5. Monitoring and Continuous Improvement
The implementation of machine learning is not a one-time process. Continuous monitoring and improvement are essential to maintain effectiveness:
- Monitor Performance: Regularly track the performance of machine learning models against predefined KPIs to ensure they are delivering the desired results.
- A/B Testing: Conduct A/B testing to compare the effectiveness of different strategies or models, enabling data-driven decision-making.
- Feedback Loop: Establish a feedback mechanism to collect user input and adapt the models based on changing customer preferences and market trends.
Challenges in Implementing Machine Learning in eCommerce
While the benefits of Machine Learning in Your eCommerce Strategy are significant, there are also challenges to consider:
1. Data Quality and Availability: Poor data quality can lead to inaccurate predictions. Businesses must ensure they have access to reliable and comprehensive datasets.
2. Complexity of Implementation: Developing and deploying machine learning models requires specialized skills and expertise, which may not be readily available in-house.
3. Integration with Existing Systems: Ensuring that machine learning solutions seamlessly integrate with existing eCommerce platforms and technologies can be a complex task.
4. Cost Considerations: The costs associated with implementing machine learning solutions, including data collection, tool acquisition, and talent recruitment, can be significant.
5. Security and Privacy: Handling customer data comes with the responsibility of ensuring compliance with data protection regulations, such as GDPR and CCPA. Businesses must prioritize data security and privacy.
Case Studies of Successful Machine Learning Implementations in eCommerce
Examining successful implementations of machine learning in eCommerce can provide valuable insights and inspiration. Here are a few notable examples:
1. Amazon’s Recommendation Engine
Amazon utilizes machine learning to power its recommendation engine, which accounts for a significant portion of its sales. By analyzing user behavior, purchase history, and preferences, Amazon can provide personalized product suggestions, enhancing the customer experience and driving conversions.
2. Walmart’s Predictive Analytics
Walmart employs predictive analytics to optimize its inventory management. By analyzing historical sales data, seasonal trends, and customer preferences, Walmart can accurately forecast demand, ensuring that the right products are available at the right time.
3. eBay’s Visual Search
eBay has implemented machine learning algorithms to enhance its search functionality. Through visual search technology, customers can upload images of products they are interested in, and the system recommends similar items available for purchase on the platform.
Best Practices for Implementing Machine Learning in Your eCommerce Strategy
To maximize the benefits of machine learning in eCommerce, consider the following best practices:
1. Start Small: Begin with a specific use case or pilot project before scaling up your machine learning initiatives. This allows you to test the waters and gain insights without overwhelming your resources.
2. Focus on Data Quality: Prioritize data quality and integrity by implementing robust data management practices. Clean and accurate data is essential for effective machine learning.
3. Collaborate with Experts: Partner with AI consulting services or machine learning experts to gain insights and expertise. This can help you navigate the complexities of implementation and ensure best practices are followed.
4. Invest in Training: Provide training and resources for your team to develop the necessary skills to work with machine learning tools and technologies. This investment will pay off in the long run.
5. Foster a Data-Driven Culture: Encourage a culture of  within your organization. This will empower teams to leverage insights from machine learning to optimize their strategies.
Conclusion
Implementing Machine Learning in Your eCommerce Strategy is a powerful way to enhance customer experiences, optimize operations, and drive better results. By focusing on data collection, choosing the right tools, developing effective models, and continuously monitoring performance, businesses can harness the full potential of machine learning.
For companies looking to enhance their eCommerce capabilities through custom mobile app development and machine learning solutions, partnering with a trusted provider named Upcore Technologies can pave the way for success. With expertise in machine learning and a deep understanding of the eCommerce landscape, Upcore Technologies can help you navigate this transformative journey and achieve your business objectives.
By following the insights and strategies outlined in this blog, you can position your eCommerce business at the forefront of innovation, ensuring sustained growth and success in the competitive marketplace.