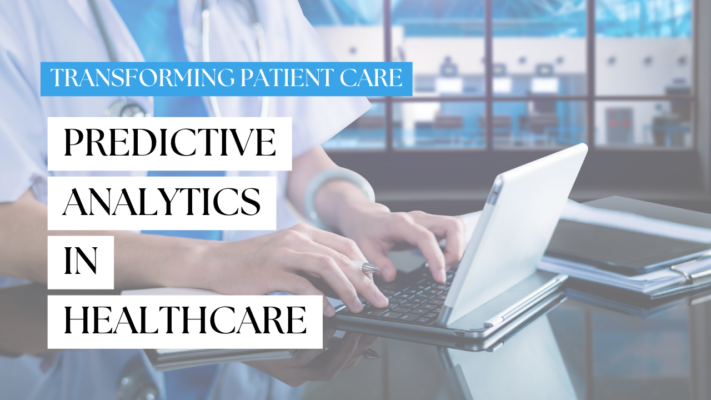
In recent years, the healthcare industry has witnessed a significant shift towards data-driven decision-making and personalized patient care. At the forefront of this transformation is predictive analytics in healthcare, a powerful tool that leverages historical and real-time data to forecast future outcomes and guide clinical decisions. This comprehensive blog post will explore the various aspects of healthcare predictive analytics, its applications, benefits, challenges, and prospects.
Understanding Predictive Analytics in Healthcare
Predictive analytics in healthcare refers to the use of statistical algorithms, machine learning techniques, and data mining to analyze large volumes of healthcare data and predict future events or trends. By combining historical patient data, clinical research, and real-time monitoring information, healthcare predictive analytics can provide valuable insights to improve patient care, optimize resource allocation, and enhance overall healthcare delivery.
The foundation of predictive analytics in healthcare lies in its ability to:
1. Identify patterns and trends in large datasets
2. Forecast future events or outcomes based on historical data
3. Assess risk factors and probabilities of various health conditions
4. Guide clinical decision-making and treatment planning
5. Optimize resource allocation and operational efficiency
Key Components of Predictive Analytics in Healthcare
To effectively implement predictive analytics in healthcare, several key components must work together:
1. Data Collection and Integration
The first step in healthcare predictive analytics is gathering and integrating data from various sources, including:
– Electronic Health Records (EHRs)
– Medical imaging systems
– Laboratory information systems
– Wearable devices and remote patient monitoring tools
– Claims and billing data
– Public health databases
2. Data Preprocessing and Cleaning
Raw healthcare data often contains errors, inconsistencies, and missing values. Data preprocessing involves:
– Removing duplicates and irrelevant information
– Handling missing data
– Standardizing data formats
– Addressing data quality issues
3. Feature Selection and Engineering
This step involves identifying the most relevant variables (features) for predicting the desired outcome. Feature engineering may include:
– Creating new variables from existing data
– Transforming variables to improve model performance
– Reducing dimensionality through techniques like Principal Component Analysis (PCA)
4. Model Development and Training
Predictive analytics in healthcare relies on various statistical and machine learning models, such as:
– Regression models (linear, logistic, etc.)
– Decision trees and random forests
– Support Vector Machines (SVM)
– Neural networks and deep learning models
– Time series analysis
5. Model Validation and Testing
To ensure the accuracy and reliability of predictive models, rigorous validation and testing are essential. This includes:
– Cross-validation techniques
– Performance metrics evaluation (e.g., accuracy, precision, recall)
– Bias and fairness assessments
6. Deployment and Integration
Once validated, predictive models are integrated into healthcare systems and workflows, enabling real-time predictions and decision support.
7. Continuous Monitoring and Improvement
Predictive analytics in healthcare requires ongoing monitoring and refinement to maintain accuracy and relevance as new data becomes available and healthcare practices evolve.
Applications of Predictive Analytics in Healthcare
Predictive analytics in healthcare has a wide range of applications across various aspects of patient care and healthcare management:
1. Disease Prevention and Early Detection
Predictive analytics in healthcare can identify individuals at high risk of developing specific diseases, enabling proactive interventions and preventive measures. Applications include:
– Predicting the onset of chronic diseases like diabetes or heart disease
– Identifying patients at risk of hospital readmission
– Forecasting potential outbreaks of infectious diseases
2. Personalized Treatment Plans
By analyzing patient data and treatment outcomes, predictive analytics in healthcare can help clinicians develop more effective, personalized treatment plans:
– Predicting patient response to specific medications or therapies
– Identifying optimal treatment sequences for complex conditions
– Forecasting potential adverse drug reactions or complications
3. Clinical Decision Support
Predictive analytics in healthcare enhances clinical decision-making by providing data-driven insights and recommendations:
– Suggesting appropriate diagnostic tests based on patient symptoms and history
– Predicting the likelihood of specific diagnoses
– Recommending evidence-based treatment options
4. Resource Allocation and Capacity Planning
Healthcare organizations can optimize resource utilization and improve operational efficiency through predictive analytics:
– Forecasting patient admission rates and length of stay
– Predicting staffing needs based on anticipated patient volume
– Optimizing inventory management for medical supplies and pharmaceuticals
5. Population Health Management
Predictive analytics in healthcare supports population health initiatives by identifying trends and risk factors across patient populations:
– Predicting the spread of infectious diseases
– Identifying high-risk patient groups for targeted interventions
– Assessing the impact of public health programs and policies
6. Fraud Detection and Prevention
Healthcare organizations can use predictive analytics to identify potential fraud and abuse in claims and billing:
– Detecting unusual billing patterns or outliers
– Predicting the likelihood of fraudulent claims
– Identifying providers or patients with suspicious activity patterns
7. Patient Engagement and Adherence
Predictive analytics in healthcare can help improve patient engagement and treatment adherence:
– Predicting the likelihood of medication non-adherence
– Identifying patients at risk of missing appointments
– Recommending personalized interventions to improve patient engagement
Benefits of Predictive Analytics in Healthcare
The adoption of healthcare predictive analytics offers numerous benefits to patients, healthcare providers, and the healthcare system as a whole:
1. Improved Patient Outcomes
By enabling early intervention and personalized treatment plans, healthcare predictive analytics can lead to better patient outcomes and reduced complications.
2. Enhanced Preventive Care
Predictive analytics helps identify high-risk individuals, allowing for proactive measures to prevent the onset or progression of diseases.
3. Reduced Healthcare Costs
By optimizing resource allocation and preventing unnecessary hospitalizations, healthcare predictive analytics can significantly reduce healthcare costs.
4. Increased Operational Efficiency
Healthcare organizations can improve their operational efficiency through better capacity planning and resource management.
5. Data-Driven Decision Making
Predictive analytics provides clinicians and administrators with data-driven insights to support more informed decision-making.
6. Personalized Patient Care
By analyzing individual patient data, predictive analytics enables more personalized and targeted healthcare interventions.
7. Improved Population Health
Predictive analytics in healthcare supports population health management initiatives by identifying trends and risk factors across patient groups.
8. Enhanced Patient Engagement
By predicting patient behaviour and preferences, healthcare providers can develop more effective strategies for patient engagement and education.
Challenges
While the potential benefits of healthcare predictive analytics are significant, several challenges must be addressed for successful implementation:
1. Data Quality and Standardization
Healthcare data often suffers from inconsistencies, missing values, and lack of standardization across different systems and organizations. Ensuring high-quality, standardized data is crucial for accurate healthcare predictive analytics.
2. Data Privacy and Security
Healthcare data is highly sensitive, and organizations must comply with strict regulations like HIPAA. Implementing robust security measures and ensuring patient privacy is essential when using healthcare predictive analytics.
3. Integration with Existing Systems
Integrating predictive analytics tools with existing healthcare IT systems and workflows can be complex and time-consuming.
4. Clinician Adoption and Trust
Healthcare professionals may be hesitant to rely on predictive models for decision-making. Building trust in healthcare predictive analytics requires transparency, validation, and education.
5. Ethical Considerations
The use of healthcare predictive analytics raises ethical questions about fairness, bias, and the potential for algorithmic discrimination.
6. Model Interpretability
Many advanced predictive models, such as deep learning algorithms, can be difficult to interpret. Ensuring model transparency and explainability is crucial for clinical adoption.
7. Regulatory Compliance
Predictive analytics in healthcare must comply with various regulations and guidelines governing the use of patient data and clinical decision-support tools.
8. Cost and Resource Requirements
Implementing healthcare predictive analytics requires significant investment in technology, infrastructure, and skilled personnel.
Best Practices for Implementing Predictive Analytics
To maximize the benefits of predictive analytics in healthcare while addressing the challenges, organizations should follow these best practices:
1. Establish Clear Objectives
Define specific goals and use cases for healthcare predictive analytics, aligned with organizational priorities and patient needs.
2. Ensure Data Quality and Governance
Implement robust data governance practices to ensure the quality, consistency, and security of healthcare data used in predictive analytics.
3. Foster Interdisciplinary Collaboration
Encourage collaboration between clinicians, data scientists, IT professionals, and administrators to develop effective predictive analytics solutions.
4. Prioritize Model Transparency and Interpretability
Choose predictive models that provide clear explanations for their predictions, enabling clinicians to understand and trust the results.
5. Validate Models Rigorously
Implement thorough validation processes to ensure the accuracy, reliability, and generalizability of predictive models across diverse patient populations.
6. Integrate with Clinical Workflows
Design predictive analytics tools that seamlessly integrate with existing clinical workflows and electronic health record systems.
7. Provide Ongoing Training and Support
Offer comprehensive training and support to healthcare professionals on the use and interpretation of predictive analytics tools.
8. Monitor and Evaluate Performance
Continuously monitor the performance of predictive models and evaluate their impact on patient outcomes and operational efficiency.
9. Address Ethical and Legal Considerations
Develop clear guidelines and policies for the ethical use of healthcare predictive analytics, ensuring compliance with relevant regulations and standards.
10. Embrace Continuous Improvement
Regularly update and refine predictive models based on new data, emerging research, and evolving healthcare practices.
Future Trends of Healthcare Predictive Analytics
As technology continues to advance, several trends are shaping the future of healthcare predictive analytics:
1. Artificial Intelligence and Deep Learning
The integration of advanced AI and deep learning techniques will enhance the accuracy and capabilities of healthcare predictive analytics, enabling more complex and nuanced predictions.
2. Real-time Predictive Analytics
Advances in computing power and data processing will enable real-time predictive analytics, allowing for immediate insights and interventions at the point of care.
3. Precision Medicine
Predictive analytics will play a crucial role in advancing precision medicine, leveraging genetic, environmental, and lifestyle data to predict individual patient responses to treatments.
4. Internet of Medical Things (IoMT)
The proliferation of connected medical devices and wearables will provide a wealth of real-time patient data, enhancing the predictive capabilities of healthcare analytics.
5. Natural Language Processing (NLP)
Advancements in Natural Language Processing will enable the extraction of valuable insights from unstructured clinical notes and medical literature, enriching predictive models.
6. Federated Learning
This emerging technique allows for the development of predictive models across multiple healthcare organizations without sharing sensitive patient data, addressing privacy concerns.
7. Explainable AI
As the complexity of predictive models increases, there will be a growing focus on developing explainable AI techniques to ensure transparency and trust in healthcare predictions.
8. Edge Computing
The integration of edge computing in healthcare devices will enable faster, more efficient predictive analytics at the point of care, reducing latency and improving real-time decision support.
9. Blockchain for Data Security
Blockchain technology may be leveraged to enhance the security and integrity of healthcare data used in predictive analytics, addressing concerns about data privacy and tampering.
10. Integration with Virtual and Augmented Reality
Predictive analytics insights may be visualized and presented through VR and AR technologies, enhancing clinical decision-making and patient education.
Conclusion
Healthcare Predictive Analytics represents a powerful tool for transforming patient care, improving outcomes, and optimizing healthcare delivery. By leveraging advanced statistical techniques, machine learning challenges and algorithms, healthcare organizations can unlock valuable insights from their vast data repositories, enabling more proactive, personalized, and efficient care.
As the field of predictive analytics in healthcare continues to evolve, it promises to revolutionize various aspects of healthcare, from disease prevention and personalized treatment to resource allocation and population health management. However, successful implementation requires addressing challenges related to data quality, privacy, integration, and ethical considerations.
Healthcare organizations that embrace predictive analytics and follow best practices for implementation will be well-positioned to deliver higher-quality care, improve patient outcomes, and thrive in an increasingly data-driven healthcare landscape.
To learn more about how your healthcare organization can leverage the power of predictive analytics and artificial intelligence to transform patient care, visit Upcore Technologies, a leading AI development agency. Our team of experts can help you develop and implement cutting-edge predictive analytics solutions tailored to your specific healthcare needs, ensuring that you stay at the forefront of innovation in patient care and healthcare management.